с кодом Python from sklearnsklearn. import StandardScaler
from sklearn.model_selection import train_test_splittrain_test_split
from sklearn.metrics import accuracy_scoreaccuracy_score
import numpy as np
import numpy.random as r
import matplotlib.pyplot as plt
def convert_y_to_vect(y):
y_vect = np.zeros((len(y), 10))
for i in range(len(y)):
y_vect[i, y[i]] = 1
return y_vect
def f(x):
return 1 / (1 + np.exp(-x))
def f_deriv(x):
return f(x) * (1 - f(x))
def setup_and_init_weights(nn_structure):
W = {}
b = {}
for l in range(1, len(nn_structure)):
W[l] = r.random_sample((nn_structure[l], nn_structure[l-1]))
b[l] = r.random_sample((nn_structure[l],))
return W, b
def init_tri_values(nn_structure):
tri_W = {}
tri_b = {}
for l in range(1, len(nn_structure)):
tri_W[l] = np.zeros((nn_structure[l], nn_structure[l-1]))
tri_b[l] = np.zeros((nn_structure[l],))
return tri_W, tri_b
# Далее запустим процесс прямого распространения через нейронную сеть:
def feed_forward(x, W, b):
h = {1: x}
z = {}
#Если первый слой, то весами является x, в противном случае
#Это выход из последнего слоя
for l in range(1, len(W) + 1):
# if it is the first layer, then the input into the weights is x, otherwise,
# it is the output from the last layer
if l == 1:
node_in = x
else:
node_in = h[l]
z[l+1] = W[l].dot(node_in) + b[l] # z^(l+1) = W^(l)*h^(l) + b^(l)
h[l+1] = f(z[l+1]) # h^(l) = f(z^(l))
return h, z
def calculate_out_layer_delta(y, h_out, z_out):
# delta^(nl) = -(y_i - h_i^(nl)) * f'(z_i^(nl))
return -(y-h_out) * f_deriv(z_out)
def calculate_hidden_delta(delta_plus_1, w_l, z_l):
# delta^(l) = (transpose(W^(l)) * delta^(l+1)) * f'(z^(l))
return np.dot(np.transpose(w_l), delta_plus_1) * f_deriv(z_l)
def train_nn(nn_structure, X, y, iter_num=3000, alpha=0.25):
W, b = setup_and_init_weights(nn_structure)
cnt = 0
m = len(y)
avg_cost_func = []
print('Starting gradient descent for {} iterations'.format(iter_num))
while cnt < iter_num:
if cnt%1000 == 0:
print('Iteration {} of {}'.format(cnt, iter_num))
tri_W, tri_b = init_tri_values(nn_structure)
avg_cost = 0
for i in range(len(y)):
delta = {}
# perform the feed forward pass and return the stored h and z values, to be used in the
# gradient descent step
h, z = feed_forward(X[i, :], W, b)
# loop from nl-1 to 1 backpropagating the errors
for l in range(len(nn_structure), 0, -1):
if l == len(nn_structure):
delta[l] = calculate_out_layer_delta(y[i,:], h[l], z[l])
avg_cost += np.linalg.norm((y[i,:]-h[l]))
else:
if l >> 1:
delta[l] = calculate_hidden_delta(delta[l+1], W[l], z[l])
# triW^(l) = triW^(l) + delta^(l+1) * transpose(h^(l))
tri_W[l] += np.dot(delta[l+1][:,np.newaxis], np.transpose(h[l][:,np.newaxis]))
# trib^(l) = trib^(l) + delta^(l+1)
tri_b[l] += delta[l+1]
# perform the gradient descent step for the weights in each layer
# запускает градиентный спуск для весов в каждом слое
for l in range(len(nn_structure) - 1, 0, -1):
W[l] += -alpha * (1.0/m * tri_W[l])
b[l] += -alpha * (1.0/m * tri_b[l])
# завершает расчеты общей оценки
avg_cost = 1,0/m * avg_cost
avg_cost_func.append(avg_cost)
cnt += 1
return W, b, avg_cost_func
# return W, b, avg_cost_func = train_nn(nn_structure, X_train, y_v_train)
def predict_y(W, b, X, n_layers):
m = X.shape[0]
y = np.zeros((m,))
for i in range(m):
h, z = feed_forward(X[i, :], W, b)
y[i] = np.argmax(h[n_layers])
return y
if __name__ == "__main__":
# load data and scale
digits = load_digits()
X_scale = StandardScaler()
X = X_scale.fit_transform(digits.data)
y = digits.target
X_train, X_test, y_train, y_test = train_test_split(X, y, test_size=0.4)
# convert digits to vectors
y_v_train = convert_y_to_vect(y_train)
y_v_test = convert_y_to_vect(y_test)
# setup the NN structure
nn_structure = [64, 30, 10]
# train the NN
W, b, avg_cost_func = train_nn(nn_structure, X_train, y_v_train)
# plot the avg_cost_func
plt.plot(avg_cost_func)
plt.ylabel('Average J')
plt.xlabel('Iteration number')
plt.show()
# get the prediction accuracy and print
y_pred = predict_y(W, b, X_test, 3)
print('Prediction accuracy is {}%'.format(accuracy_score(y_test, y_pred) * 100))
103
270
Ответы на вопрос:
Реши свою проблему, спроси otvet5GPT
-
Быстро
Мгновенный ответ на твой вопрос -
Точно
Бот обладает знаниями во всех сферах -
Бесплатно
Задай вопрос и получи ответ бесплатно
Популярно: Информатика
-
KOJIOCOK15.02.2021 12:47
-
zharkovaaa113331.01.2023 15:08
-
linayaryshkina03.04.2022 11:14
-
1337lolkek24.02.2022 11:48
-
romamuver30.06.2023 12:54
-
тимур62406.11.2020 22:09
-
nikzarubenko18.04.2022 18:22
-
Kisa2Mary11.06.2023 22:10
-
4344676494912.09.2021 00:55
-
малика23206.10.2020 06:58
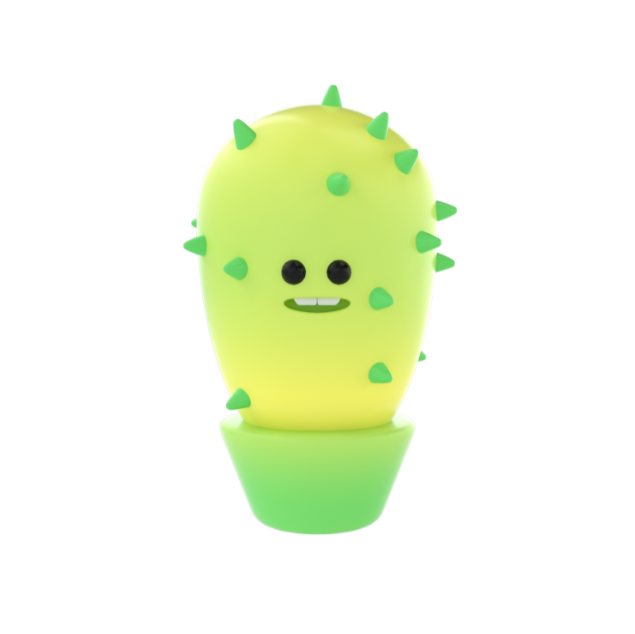
Есть вопросы?
-
Как otvet5GPT работает?
otvet5GPT использует большую языковую модель вместе с базой данных GPT для обеспечения высококачественных образовательных результатов. otvet5GPT действует как доступный академический ресурс вне класса. -
Сколько это стоит?
Проект находиться на стадии тестирования и все услуги бесплатны. -
Могу ли я использовать otvet5GPT в школе?
Конечно! Нейросеть может помочь вам делать конспекты лекций, придумывать идеи в классе и многое другое! -
В чем отличия от ChatGPT?
otvet5GPT черпает академические источники из собственной базы данных и предназначен специально для студентов. otvet5GPT также адаптируется к вашему стилю письма, предоставляя ряд образовательных инструментов, предназначенных для улучшения обучения.